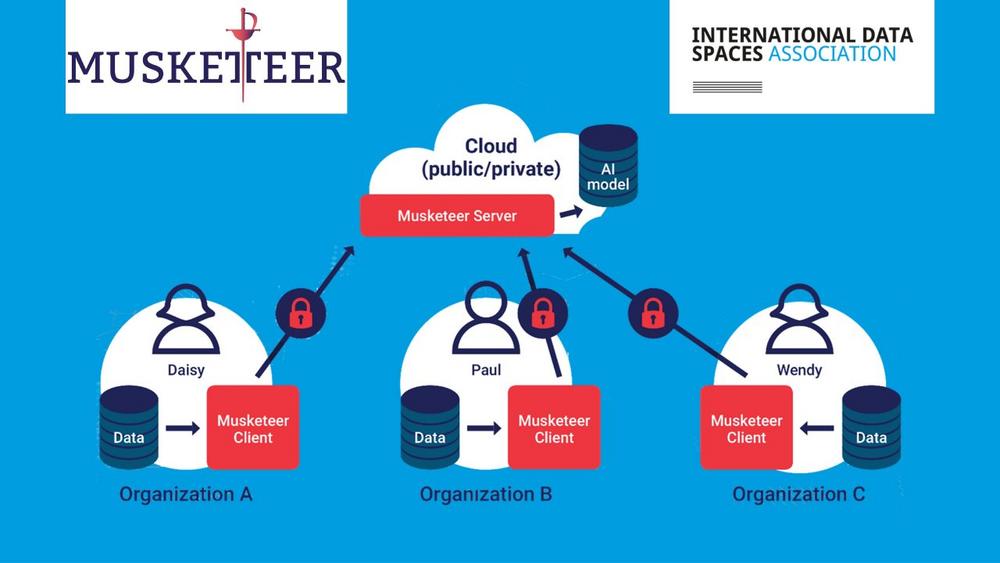
Federated learning to serve smart manufacturing process improvement while retaining control of confidential data
The presence of a huge number of machines in industrial automation factories and the elevated cost of downtime, produce large expenses for production line maintenance. Getting a more accurate evaluation of robot performance helps to avoid damaging the production capacity contingently (by 5 to 20% in certain cases)[1]. But collecting data from different factories to build powerful AI tools can raise privacy issues. Data used to train AI models can be sensitive company data but also lead to personal data concerns (e.g. data can include information about operators working at the plant). The MUSKETEER platform offers a solution to tackle these issues mixing privacy preserving technologies (federated machine learning, etc.) while respecting sovereignty of the stakeholders as defined by IDSA standard[2].
The first project release concerns the MUSKETEER client package. It describes how to interact with the IBM MUSKETEER cloud platform for federated machine learning. Developers learn how to design and run federated machine learning algorithms on the platform:
https://github.com/IBM/Musketeer-Client
This package, which is tested in a manufacturing use case, is helping to solve complex challenges for the automotive sector:
- Improving the welding quality assessment to develop predictive maintenance for robots while increasing product safety at the same time
- Training a welding quality assessment algorithm on large datasets from multiple factories
To find out more about this story and discover the complete scenario along with user testimonials:
https://musketeer.eu/publications/
MUSKETEER project has received funding from the European Union’s Horizon 2020 research and innovation programme under grant agreement No 824988.
[1] https://www2.deloitte.com/... and operations/us cons predictivemaintenance.pdf
[2] https://www.internationaldataspaces.org/… content/uploads/2019/03/IDS Reference Architecture Model 3.0.pdf
The International Data Spaces Association (IDSA) has defined a reference architecture and a global standard for creating and operating virtual data spaces. The IDS Architecture is based on commonly recognized data governance models facilitating secure exchange and easy linkage of data within business ecosystems. IDSA is a non-profit association with currently more than 120 members from numerous companies, organizations and research institutes across 20 countries.
International Data Spaces e. V.
Emil-Figge-Str. 80
44227 Dortmund
Telefon: +49 (231) 70096-501
http://www.internationaldataspaces.org
EU Programs & Partnerships Manager, IBM
Telefon: 353-87-693-6134
E-Mail: wgal@ie.ibm.com

